Preparing the data for OLAP
The global issues out of the way, the enterprise must begin to focus on the granular design issues. The data in the data warehouse must be prepared for the application of Online Analytical Processing (OLAP) solutions and such preparations will be driven by the business needs of the enterprise.
Designing the Dimensional Model
User requirements and data realities drive the design of the dimensional model. The grain of detail and the type of facts to be included are decided by the business needs and the type of analytics and reports the end user wishes to generate. Maintenance issues and scalability issues determine the type of model created.
Central to the dimensional model are the Dimension tables which are linked to Fact tables. Dimension tables are tables which encapsulate the attributes associated with a particular subject into a table. A dimension table may relate to a customer, a product or a geographical region. Dimension tables have three kinds of fields—a primary key field, hierarchy level field and attribute fields. Dimension tables are linked to fact tables using the primary key of the dimension table and the foreign key of the fact table. Dimension tables are relatively small tables compared to fact tables. A dimension table may be used in multiple places if the data warehouse contains multiple fact tables that link to the dimension table. Such tables are known as Conforming dimensions. Use of conforming dimensions is critical to the design of the data warehouse.
Dimension tables define a dimension. A dimension is hierarchical and the nature of the hierarchy is determined by the needs of the group requiring the dimension. For example the time dimension may contain the day, week, month, year and quarter as attributes. A two dimension hierarchy may have customer and area as a dimension.
A dimension may also contain multiple hierarchies. For instance a time dimension may contain a calendar year and a fiscal year as hierarchies. A multiple hierarchy dimension in the customer dimension table would be area dimension, customer dimension and product dimension. This will be represented as a three dimensional model of data. This figure represents a three dimensional hierarchy
As a safety measure most dimension tables provide for an omnibus attribute called “all” such as “all customers”, “all products”. This is an artificial
category used for grouping the first level category of the dimension and permits summarization of fact data to a single number for a dimension. A hierarchy may be balanced, unbalanced, ragged, or composed of parent child relationships. We will be dealing with these attributes later in the series.
Surrogate keys are keys defined and maintained in the data warehouse to uniquely identify records in the dimension. GUID(globally unique numbers) and Identity keys are sometimes used in data derived from distributed sources to identify them.
Fact Tables
A fact table contains business event details and addresses unique business problems, process and user needs. Fact tables are very large containing millions of rows and consuming hundreds of gigabytes of space. Since dimension tables contain descriptions of facts, the fact table can be reduced to columns for dimension foreign keys and numeric fact values.
Data warehouses may contain multiple fact tables. Each fact table may relate to one particular user requirement or business need. The fact tables are related to the dimension tables relating to the business function in schemas known as star or snowflake schemas. Such business specific schemas may be part of the central data warehouse or implemented in separate data marts.
Very large fact tables are partitioned physically for ease of implementation and design considerations. The partitions are usually on a single dimension-mostly time dimension as the data in the data warehouse is historical in nature. The OLAP cubes that are developed using the partitioned fact tables are also partitioned to match the partition in the fact table.
Measures are values that quantify facts and are numeric. Measures are usually additive along dimensions. For example Quantity by customer, product and time results in a meaningful value. However non-additive values also can exist along dimensions. The quantity on hand measure is a non-additive value. Calculated measures are measures that result from applying a function to one or more measures. An example of this measure is the result of a multiplying price with product quantity.
The logical model of a fact table contains a foreign key column for the primary keys of each dimension. The combination of these foreign keys defines the primary key for the fact table. The type of composite key defined will be determined by the type of partitions required, the load performance needed etc.
The fact table resolves many-to-many relationships between dimensions because the dimension tables join through the fact table.
The granularity of the fact table is determined by the fact content columns that have been identified. Granularity is the measure of the level of detail addressed by the individual entries in the fact table.
Design of the Dimensional Model schemas – The primary characteristic of a dimensional model is set of dimension tables connected to a fact table through the foreign keys in the fact table and the primary keys in the dimension tables. The fact table and the dimension tables in a database can be linked directly or indirectly. When multiple dimension tables are linked to a fact table a Star Schema is formed. When some tables are linked directly to a fact table while others are linked indirectly by linking to the linked dimension table a Snowflake Schema is realized.
The Star Schema
The star schema is created when all the dimension tables directly link to the fact table. This is graphically represented as under.
The star schema dimensional model.
Since the graphical representation resembles a star it is called a star schema. It must be noted that the foreign keys in the fact table link to the primary key of the dimension table. This sample provides the star schema for a sales_ fact for the year 1998. The dimensions created are Store, Customer, Product_class and time_by_day. The Product table links to the product_class table through the primary key and indirectly to the fact table. The fact table contains foreign keys that link to the dimension tables.
The Snowflake Schema
The snowflake schema is a schema in which the fact table is indirectly linked to a number of dimension tables. The dimension tables are normalized to remove redundant data and partitioned into a number of dimension tables for ease of maintenance. An example of the snowflake schema is the splitting of the Product dimension into the product_category dimension and product_manufacturer dimension..
|
|
|
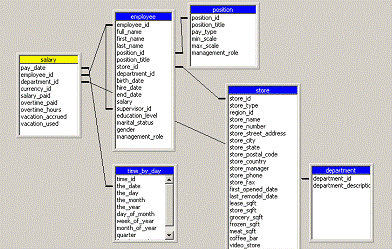
Sometimes the star schema is optimized by combining all the dimension tables into a single table. The resultant table is known as a Cartesian product. The optimized star table consists of the fact table and the combined dimension tables. The advantage of this combination is that the queries do not have to perform join operations. The only join operation would be between the fact table and the combined dimension table.
Data warehouse structures must be designed to accommodate current and future business needs of the enterprise. It must be scalable enough to accommodate additional demands with minimum of change to the fundamental design of the warehouse. Dimensional modeling has the advantage of being scaleable. It can be expanded by addition of records to dimension tables. New dimensions and schemas can be added with ease. Existing dimensions can be used with the new dimensions without modification to maintain conformity throughout the entire warehouse. If granularity is to be added, dimension tables can be partitioned to granular levels for drilling down operations on the data. OLAP cubes can be extended to accommodate new dimensions by extending their schemas and reprocessing or creating new virtual cubes that contain new dimensions. Existing cubes can be incorporated without modification.
{mospagebreak}
Design of the Relational Database and OLAP Cubes and Data mining tools The next phase in the data warehouse design is the design of the relational database and the OLAP cubes. With this the design phase moves into a more granular level of designing surrogate keys, primary and foreign key relationships, Views, indexes, partitions of fact tables. OLAP cubes are created to support the query needs of end users.
Dimension tables are indexed on their primary key. These are generally surrogate keys created in the data warehouse tables. The fact table must have a unique index on the primary key. Indexes on primary keys can be clustered or non clustered depending on the volume of schemas being maintained in the data warehouse.
Views are created for users who need direct access to data in the data warehouse relational database. Indexed views are used to improve performance of user queries that access data through views. View definitions create column and table names that make sense to the end user.
The applications that support data analysis are constructed in this phase of the design. OLAP cubes design requirements are then defined by the parameters set in the dimensional model of the data warehouse. Cubes provide fast access to data in a data warehouse. A cube has been defined as a multidimensional data structure with defined dimensions and measures and constructed out of a subset of data of the data warehouse.
Though data mining does not determine the wrap and hoof of the data warehouse design, data mining is the final manifestation of the data warehouse. Data mining uses sophisticated algorithms to analyze data and create models that represent information about the data. Data mining models are used to predict characteristics of new data or to identify groups of data entities.
The data warehouse reflects the business model of the enterprise and therefore an essential element of data warehousing design is the creation and management of metadata. Metadata is data about data. Many different kinds of metadata are to be created and managed. Administrative metadata includes the information necessary for setting up and using the warehouse. Business metadata includes business terms and definitions, ownership details and changing policy details. Operational metadata stores information that is collected during the operation of the warehouse such as the lineage of migrated and transformed data, the currency of data etc.
It is important to build in adequate security measures into the data warehouse system. The organizations security policy will impact on who can access or view data. Security policies will demand the creation of multiple copies of the data or creation of data views and possible classification of data on basis of security codes.
Closely allied to the security considerations is the backup and disaster recovery plans for the data warehouse.
The key to successful data warehousing is data design as defined by end users. Business users know what they want and they focus upon it. They will determine what data will be needed, where to locate it, how it can harnessed, what dimensions are to be created and what output is expected. The remaining tasks are a natural outcome of the business needs. Therefore, the best data warehouse design naturally and gracefully flows out of the business requirements of the end user.